Maylinda, Eka Christy (2025) PREDIKSI PELANGGAN CHURN MENGGUNAKAN ALGORITMA RANDOM FOREST DENGAN SEGMENTASI K-MEANS DALAM INDUSTRI RETAIL OTOMOTIF (Studi Kasus: KALLA TOYOTA). Skripsi thesis, Universitas Hasanuddin Makassar.
![[thumbnail of D121201050_skripsi_16-10-2024 bab 1-2.pdf]](/style/images/fileicons/text.png)
D121201050_skripsi_16-10-2024 bab 1-2.pdf
Download (3MB)
![[thumbnail of D121201050_skripsi_16-10-2024 cover1.jpg]](/44463/2.hassmallThumbnailVersion/D121201050_skripsi_16-10-2024%20cover1.jpg)
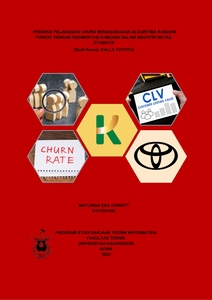
D121201050_skripsi_16-10-2024 cover1.jpg
Download (374kB) | Preview
![[thumbnail of D121201050_skripsi_16-10-2024 dp.pdf]](/style/images/fileicons/text.png)
D121201050_skripsi_16-10-2024 dp.pdf
Download (1MB)
![[thumbnail of D121201050_skripsi_16-10-2024.pdf]](/style/images/fileicons/text.png)
D121201050_skripsi_16-10-2024.pdf
Restricted to Repository staff only until 27 February 2027.
Download (6MB)
Abstract (Abstrak)
Background. Kalla Toyota, a company that has been operating for nearly 70 years and plays a significant role in the automotive industry, has yet to efficiently implement customer segmentation in its service workshops. Effective segmentation has been proven to enhance customer loyalty and reduce churn, as recognized by 90% of industry professionals. Aim. This study aims to identify customer segments at Kalla Toyota using the K-Means algorithm and predict customer churn classes using the Random Forest algorithm. The research is divided into two stages: 1) Customer segmentation using CLTV (Customer Lifetime Value), a metric that measures the financial value of the long-term relationship between a business and its customers, and K-Means; 2) Customer churn prediction based on segmentation using Random Forest. Results. The customer segmentation process identified five clusters: 1) High Churn Potential; 2) New Customers; 3) High Loyalty Potential; 4) Mid-to-High Churn; 5) Mid-to-High Loyalty. A Silhouette Score of 0.53 supports the validity of the segmentation, while the customer class prediction using the Random Forest model achieved an accuracy of 87%. Conclusion. The segmentation using K-Means and customer churn prediction with Random Forest effectively identify potential churn risks, allowing the company to optimize its retention strategies.
Item Type: | Thesis (Skripsi) |
---|---|
Subjects: | T Technology > T Technology (General) |
Divisions (Program Studi): | Fakultas Teknik > Teknik Informatika |
Depositing User: | Unnamed user with username pkl2 |
Date Deposited: | 07 May 2025 01:52 |
Last Modified: | 07 May 2025 01:52 |
URI: | http://repository.unhas.ac.id:443/id/eprint/44463 |